Neural Compression
Machine Learning and Compression Workshop @ NeurIPS 2024
Workshop Info
Date & location: Sun Dec 15, 2024. West Meeting Room 211-214
Schedule and NeurIPS virtual site: https://neurips.cc/virtual/2024/workshop/84753
Accepted papers: OpenReview
Virtual posters and presentations: Google Drive
Awards
Best paper award🏆
Transformers Learn to Compress Variable-order Markov Chains in-Context. Ruida Zhou, Chao Tian, Suhas Diggavi
Oral presentations🏆
Transformers Learn to Compress Variable-order Markov Chains in-Context. Ruida Zhou, Chao Tian, Suhas Diggavi
Getting free Bits Back from Rotational Symmetries in LLMs. Jiajun He, Gergely Flamich, José Miguel Hernández-Lobato
Prechastic Coding: An Alternative Approach to Neural Network Description Lengths. Paris Dominic Louis Flood, Pietro Lio
An Information Theory of Compute-Optimal Size Scaling, Emergence, and Plateaus in Language Models. Anuj K. Nayak, Lav R. Varshney
Spotlight presentations🏆
Interpretability as Compression: Reconsidering SAE Explanations of Neural Activations. Kola Ayonrinde, Michael T Pearce, Lee Sharkey
Diffusion Models With Learned Adaptive Noise. Subham Sekhar Sahoo, Aaron Gokaslan, Christopher De Sa, Volodymyr Kuleshov
The Rate-Distortion-Perception Trade-Off with Algorithmic Realism. Yassine Hamdi, Aaron B. Wagner, Deniz Gunduz
The Trichromatic Strong Lottery Ticket Hypothesis: Neural Compression With Three Primary Supermasks. Ángel López García-Arias, Yasuyuki Okoshi, Hikari Otsuka, Daiki Chijiwa, Yasuhiro Fujiwara, Susumu Takeuchi, Masato Motomura
Best reviewer award🏆 goes to Thanh-Dung Le.
Congratulations to all the award winners!
Call for Papers
The workshop solicits original research in the intersection of machine learning, data/model compression, and more broadly information theory.
Machine learning and compression have been described as “two sides of the same coin”, and the exponential amount of data being generated in diverse domains underscores the need for improved compression as well as efficient AI systems. Leveraging deep generative models, recent machine learning-based methods have set new benchmarks for compressing images, videos, and audio. Despite these advances, many open problems remain, such as computational efficiency, performance guarantees, and channel simulation. Parallel advances in large-scale foundation models further spurred research in efficient AI techniques such as model compression and distillation. This workshop aims to bring together researchers in machine learning, data/model compression, and information theory. It will focus on enhancing compression techniques, accelerating large model training and inference, exploring theoretical limits, and integrating information-theoretic principles to improve learning and generalization. By bridging disciplines, we seek to catalyze the next generation of scalable, efficient information-processing systems.
Topics of interest include, but are not limited to,
- Improvements in learning-based techniques for compressing data, model weights, implicit/learned representations of signals, and emerging data modalities.
- Accelerating training and inference for large foundation models, potentially in distributed settings.
- Theoretical understanding of neural compression methods, including but not limited to fundamental information-theoretic limits, perceptual/realism metrics, distributed compression and compression without quantization.
- Understanding/improving learning and generalization via compression and information-theoretic principles.
- Information-theoretic aspects of unsupervised learning and representation learning.
Call for Reviewers
Please fill out this Google form if you are interested in reviewing for the workshop. Best reviewer wins free registration for the full conference!
Important Dates
- Submission deadline:
Sept 30, 2024 (Anywhere on Earth) - Notification date:
Oct 9, 2024 - Workshop date: Dec 15, 2024
Submission Instructions
Submission website: OpenReview
We solicit short workshop paper submissions of up to 4 pages 6 pages + unlimited references/appendices. Please format submissions in NeurIPS style. Submissions will be double blind: reviewers cannot see author names when conducting reviews, and authors cannot see reviewer names.
All accepted papers are expected to be presented as posters at the poster session, and published via Openreview after the workshop. Some accepted papers will be selected as contributed/spotlight talks.
This workshop will not have formal proceedings, so we welcome the submission of work currently under review at other archival ML venues (for example, shorter versions of main conference submissions can be submitted to our workshop concurrently). We also welcome the submission of work recently published in information theory venues (e.g. Transactions on Information Theory, ISIT, ITW) that may be of interest to an ML audience. However, we will not consider work recently published in or accepted to other archival ML venues (e.g., NeurIPS main conference) to encourage the presentation of fresh and cutting-edge results at this workshop.
Speakers
![]() |
![]() |
![]() |
![]() |
Emilien Dupont Research Scientist, DeepMind |
Ziv Goldfeld Assistant Professor, Cornell |
Ashish Khisti Professor, University of Toronto |
Sanae Lotfi PhD Student, NYU |
![]() |
|||
Ayfer Özgür Associate Professor, Stanford |
Panelists
![]() |
![]() |
![]() |
![]() |
Aaron Wagner Professor, Cornell |
Sanae Lotfi PhD Student, NYU |
Ashish Khisti Professor, University of Toronto |
Ayfer Özgür Associate Professor, Stanford |
Organizers
![]() |
![]() |
![]() |
![]() |
Yibo Yang PhD Student, UC Irvine |
Karen Ullrich Research Scientist, Meta AI |
Justus Will PhD Student, UC Irvine |
Ezgi Özyılkan PhD Student, NYU |
![]() |
![]() |
||
Elza Erkip Professor, NYU |
Stephan Mandt Associate Professor, UC Irvine |
Organizers affiliations
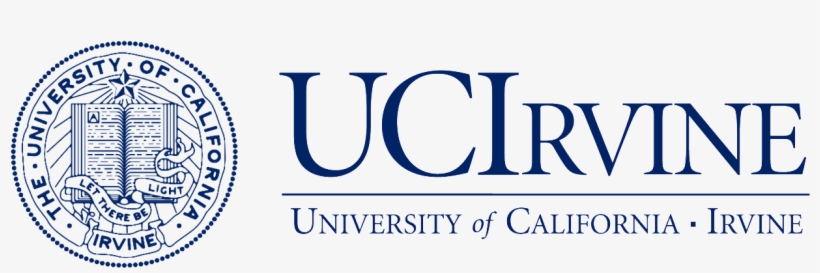
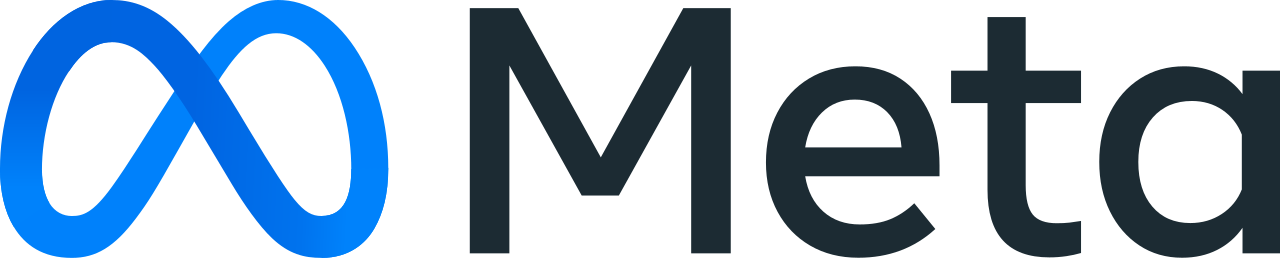
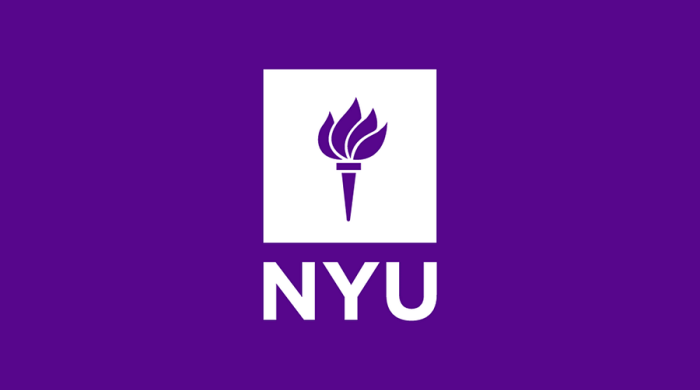