Neural Compression
Neural Compression Workshop @ ICML 2023
From Information Theory to Applications
ICML page with recording/slides: https://icml.cc/virtual/2023/workshop/21499
Accepted papers: OpenReview
Awards
Oral presentations
Neural Distributed Compressor Does Binning. Ezgi Ozyilkan, Johannes Ballé, Elza Erkip
Entropy Coding of Unordered Data Structures. Julius Kunze, Daniel Severo, Giulio Zani, Jan-Willem van de Meent, James Townsend
Neural Image Compression: Generalization, Robustness, and Spectral Bias. Kelsey Lieberman, James Diffenderfer, Charles Godfrey, Bhavya Kailkhura
Slicing Mutual Information Generalization Bounds for Neural Networks. Kimia Nadjahi, Kristjan Greenewald, Rickard Brüel Gabrielsson, Justin Solomon 🏆 Best paper award🏆
Spotlight presentations
Estimating the Rate-Distortion Function by Wasserstein Gradient Descent. Yibo Yang, Stephan Eckstein, Marcel Nutz, Stephan Mandt
Lossy Image Compression with Conditional Diffusion Model. Ruihan Yang, Stephan Mandt
NNCodec: An Open Source Software Implementation of the Neural Network Coding ISO/IEC Standard. Daniel Becking, Paul Haase, Heiner Kirchhoffer, Karsten Müller, Wojciech Samek, Detlev Marpe
On the Choice of Perception Loss Function for Learned Video Compression. Buu Phan, Sadaf Salehkalaibar, Jun Chen, Wei Yu, Ashish J Khisti
Reconstruction Distortion of Learned Image Compression with Imperceptible Perturbations. Yang Sui, Zhuohang Li, Ding Ding, Xiang Pan, Xiaozhong Xu, Shan Liu, Zhenzhong Chen
Call for Papers
The workshop solicits original research on the topic of learning-based approaches to data compression and communication.
The ubiquity of communication technology has made efficient and effective data compression an increasingly critical research area. Recent work building on deep generative models such as variational autoencoders, GANs, normalizing flows, and diffusion models has shown that machine-learning-based compression methods can significantly outperform state-of-the-art classical compression codecs for image and video data. However, there are still open questions and practical problems holding back these methods from making a major impact in real-world applications.
This workshop aims to address these issues by bringing together researchers from diverse fields including deep generative modeling, information theory, and statistics (Bayesian or otherwise). We believe that a multi-disciplinary approach to compression will lead to new synergies and result in a new generation of learnable codecs, as well as a better understanding of theoretical aspects.
Topics of interest include, but are not limited to,
- Data compression (e.g., images, video, audio) with machine learning
- Probabilistic modeling and inference for compression
- Quantization, entropy coding, and stochastic coding
- Theoretic understanding of learned compression
- Fundamental performance bounds/limits
- Learning-based approaches to information theory
- Computationally-efficient models/methods
- Perceptual metrics and image quality assessment algorithms
Important Dates
Submission deadline: May 27 11:59 PM AOE (anywhere on earth), 2023Notification date: June 19, 2023Workshop date: 9 AM - 5 PM Hawaii time (HST), July 29, 2023
Submission Instructions
Submission website: OpenReview
We solicit short workshop paper submissions of up to 4 pages + unlimited references/appendices. Please format submissions in ICML style. Submissions will be double blind: reviewers cannot see author names when conducting reviews, and authors cannot see reviewer names.
Some accepted papers will be accepted as contributed talks. All accepted posters are expected to be presented in-person at the poster session, and all papers published via Openreview after the workshop.
This workshop will not have formal proceedings, so we welcome the submission of work currently under review at other archival ML venues. We also welcome the submission of work recently published in information theory venues (e.g. Transactions on Information Theory, ISIT, ITW) that may be of interest to an ML audience. However, we will not consider work recently published in or accepted to other archival ML venues (e.g. ICML main conference).
Speakers
![]() |
![]() |
![]() |
![]() |
Johannes Ballé Research Scientist, Google |
José Miguel Hernández-Lobato Professor, Cambridge |
Hyeji Kim Assistant Professor, UT Austin |
Yan Lu Partner Research Manager, Microsoft Research Asia |
![]() |
![]() |
||
Aaron Wagner Professor, Cornell |
Tsachy Weissman Professor, Stanford |
Panelists
![]() |
![]() |
![]() |
![]() |
Ashish Khisti Professor, University of Toronto |
Ties van Rozendaal Senior Deep Learning Researcher, Qualcomm |
George Toderici Senior Staff Research Scientist, Google |
Rashmi Vinayak Assistant Professor, CMU |
Organizers
![]() |
![]() |
![]() |
![]() |
Berivan Isik PhD Student, Stanford |
Yibo Yang PhD Student, UC Irvine |
Daniel Severo PhD Student, University of Toronto and Vector Institute for AI |
Karen Ullrich Research Scientist, Meta AI |
![]() |
![]() |
||
Robert Bamler Professor, University of Tübingen |
Stephan Mandt Associate Professor, UC Irvine |
Organizers affiliations
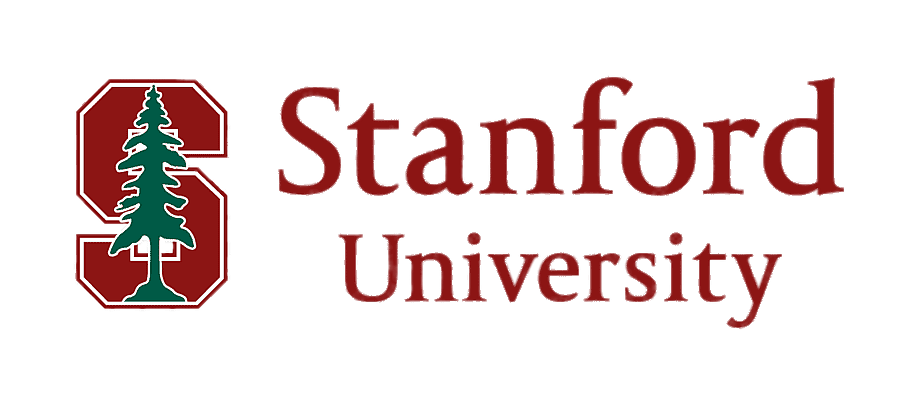
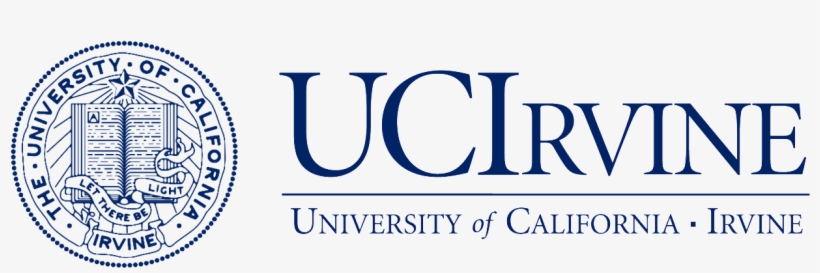
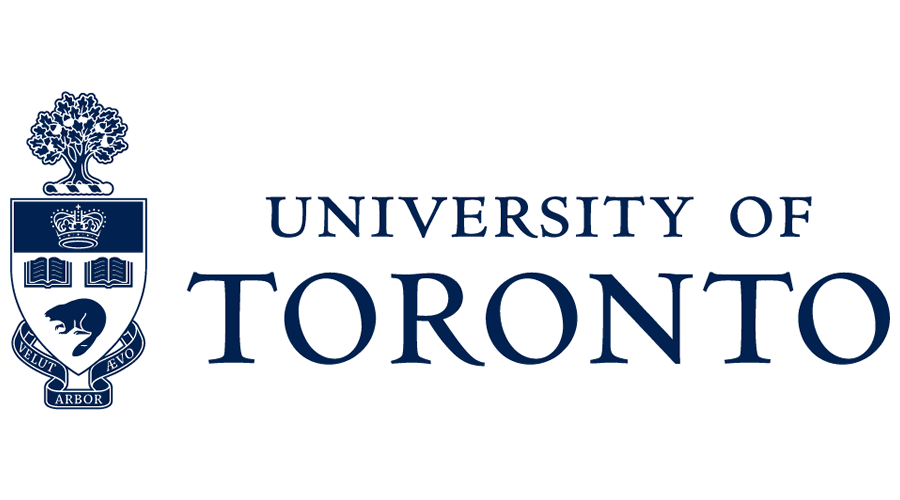
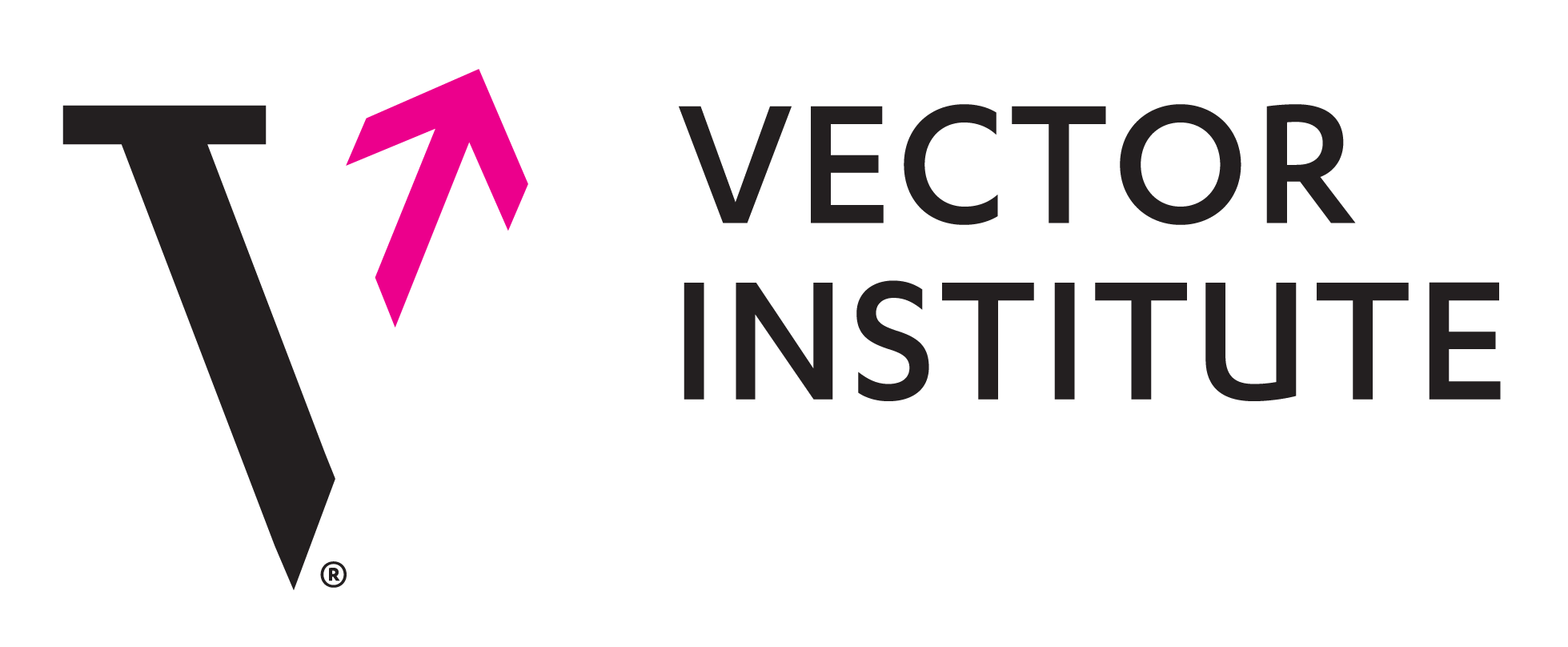
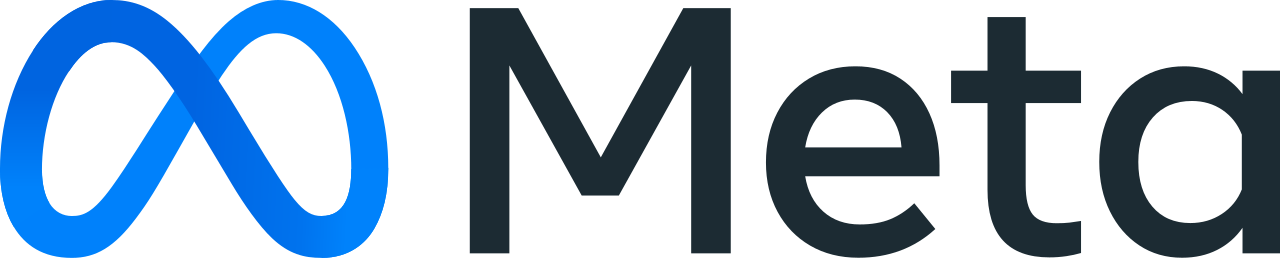
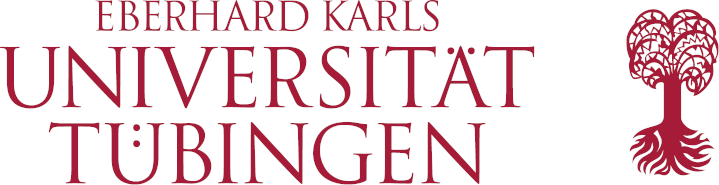